.png)
Data lake x data warehouse: which is the best option for your company?
With the advent of big data, companies are increasingly hungry for technologies to manage their immense amount of data , such as a data lake (DL) or a data warehouse (DW) .
This demand has been growing because, to extract, load and transform so much data , accessible and scalable storage is needed for teams to work with. And currently, this is only possible with a DL or a DW.
In this post, you will learn about the differences between these two technologies so you can know which one is the best option for your company . Follow along!
The differences between data lake and data warehouse
Today, there are two practical and efficient options for data storage : the data warehouse and the data lake . Both are viable solutions for implementing big data projects , but they must be evaluated on a case-by-case basis.
They present technical and conceptual differences in terms of architecture and purpose .
For example, unlike data lakes , data warehouses are mandatory elements in building large-scale big data solutions . In other words, it is virtually impossible to build a complete big data project without implementing a DW.
This does not mean that it overlaps with the data lake .
Did you find it confusing?
Let’s better explain the differences between the two using four main criteria:
- data format
- storage
- costs
- users
Check out!
1) Data format
Unlike a data warehouse , which stores only structured data , a data lake allows you to store all types of data - structured, unstructured and hybrid - in one place.
You can think of a data lake as a large “data lake” that contains information of many different types and sizes. Therefore, it is a much broader repository, which allows for additional and less restrictive analyzes than a DW, such as text searches, real-time data analysis , machine learning , etc.
2) Storage
On the one hand we have data lakes, which are huge and cheap repositories, capable of storing large amounts of structured and unstructured data. They even store raw data, that is, without loss , which can be used in the future both in a data warehouse and for direct analytical queries.
On the other side, there are data warehouses (or data marts), which are optimized for specific queries , but “lose” post-aggregation data because they are based only on structured data.
3) Costs
Storing data in a data warehouse is neither simple nor cheap. You can't just load random data into it. It is necessary to prepare, transform and structure large volumes of data . And this process is extremely costly for companies.
A data lake , on the other hand, as it has a more flexible and less rigid structure , does not require as many efforts to transform and structure data and, therefore, is cheaper.
A widely used alternative to extract the best of both solutions is the integration of a DL within a DW . This process is simpler and almost never requires structuring the data before loading it into the DW.
4) Users
Business analysts and stakeholders make up the majority of users of large data warehouses . In general, they use these solutions to extract insights from data and integrate them into strategic decision making.
Data lakes , on the other hand, are most explored by engineers and data scientists for the purpose of temporarily storing large volumes of data or conducting data experiments.
Beware the data swamp!
The ease and low cost of storage create a temptation to include any and all data generated by the company in the data lake , without organization and documentation.
When this happens, the DL can become a “data swamp” and lose its original functionality.
In other words, both the data lake and the data warehouse are complex structures that must be designed and implemented by professionals with experience in the subject .
And now, invest in a data lake or a data warehouse?
Many companies come to us with the following question: which is better, a data warehouse or a data lake?
There is no single answer to this question.
As we demonstrated throughout this post, both solutions are different and have pros and cons , so it is difficult to decide which one is the best.
The right question would actually be: what is the best approach for my company?
This is because the choice between one option or another depends on intrinsic elements of each organization, such as its size, its limitations and its objectives with big data projects .
In many cases, it is not necessary to choose just one option!
Despite the differences, data lake and data warehouse are two complementary tools that generate a lot of value when they work in sync . Therefore, we often recommend integrating the two solutions.
This happens, for example, when companies come to us to carry out big data projects , but they also need to store raw data to perform quick analytical queries .
In these cases, the initial data is stored in its raw format in the data lake and then goes through the ETL\ELT process to load and transform this information into the data warehouse for future analysis.
It has already been proven that, when both solutions operate in an integrated and harmonious manner, the potential of big data is leveraged. This makes decision-making easier and organizations obtain a series of advantages such as:
- best cost/benefit
- process optimization
- time saving
What is the next step?
To decide whether your big data solution will involve a data warehouse and a data lake, or just a DW, you need to analyze the advantages and disadvantages of each tool in relation to your business and then choose the one that is the best fit .
In practice, we know that the choice process can be complex, but that is not a problem. Our analytics team is prepared to help you overcome these challenges.
Contact us by clicking here .
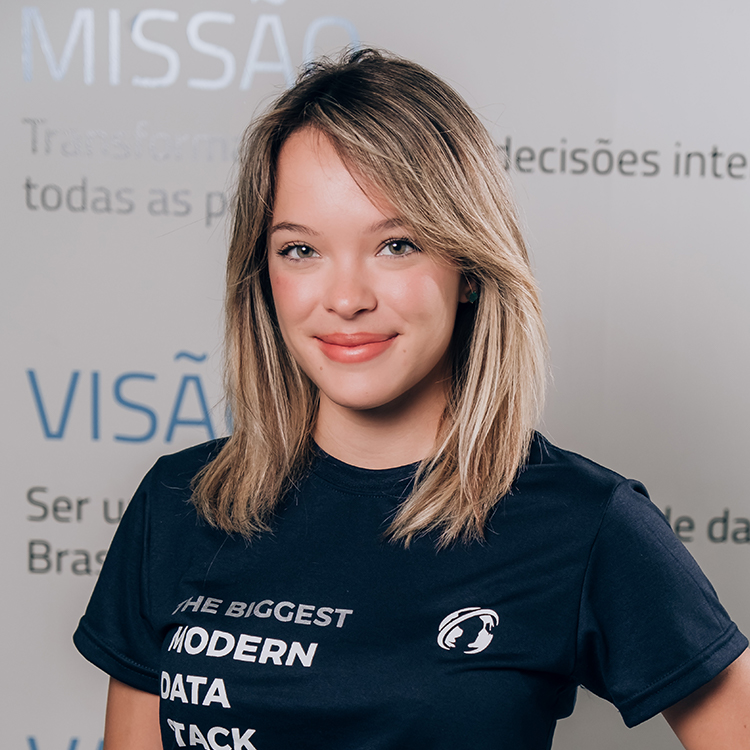
Isabela Blasi
CBDO and co-founder at Indicium